Integration of Artificial Intelligence in Radiology
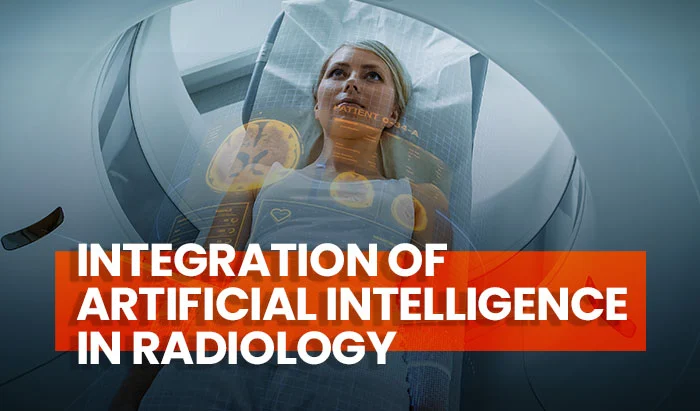
Table of Content
Artificial intelligence (AI) is an umbrella term that includes the theory and development of computer systems that perform tasks normally requiring human intelligence, such as visual perception, speech recognition, decision-making, and prediction. It is a data-driven paradigm that fits well with the technology-driven practice of modern medical imaging and, particularly, with computer vision tasks [1]. This article provides a comprehensive overview of the future role of AI in radiology as well as the role of radiologic technologists in integrating artificial intelligence into medical imaging and radiology.
According to the limited literature available, most radiographers view the integration of artificial intelligence into radiology positively as it may lead to more advanced role developments. Others feel that AI may deplete or threaten their jobs in the future. However, this notion of role depletion was not universally supported as 47% of diagnostic and 38% of therapeutic radiographers felt that AI would create new specialized/advanced roles in the future. This means that radiographers can work hand in hand with AI tools to fulfill roles that address the ongoing staff shortages [2].
Artificial Intelligence Applications in Radiology
Pre-examination assessment
Radiographers have an important role in interacting with patients before, during, and after their imaging session. Checking for patients’ identification and indications for the examination sought, as well as educating the patient about the procedure to be performed, are all part of this interaction. While direct human communication between patients and health professionals is unlikely to be replaced by AI technologies, AI systems have the potential to aid in the automated vetting of referrals and sense-checking clinical indications and the corresponding imaging modality and techniques to be used, as well as verifying patient identification records through interaction with the electronic health record.
The prospective power of AI to concurrently acquire, integrate, and synthesize knowledge and data from a variety of patient data portals exceeds that of the human rad tech and hence provides a natural environment for efficiency savings. To guarantee that patient electronic health record data is not corrupted, and AI decisions are consistent, radiographer monitoring and attention are necessary.
Examination planning
During CT and MRI studies, inaccurate patient positioning (non-isocentric) can result in reduced image quality and for CT, increased patient dose. An important aspect of the radiographer’s role is to ensure the correct patient position within the gantry or bore by using the scout view analysis (topogram). Research suggests that isocentric positioning and scout image analysis in CT, alongside MR plane and volume calculations, are areas that may be automatized by AI systems [3].
There is also the potential for intelligent systems to optimize contrast volume and injection rates based on patient factors, as well as the use of no contrast at all via synthetic contrast enhancement, embracing the idea of customized and tailored treatment [4].
Image acquisition
Selecting the correct imaging protocol based on patient presentation, clinical question, and region of interest is an important radiographer’s responsibility but evidence suggests that the choice and application of the protocol are not consistent within, or across, hospital sites or imaging modalities. Emerging research suggests that AI may make protocol selection automatable. There is also a plethora of AI-driven dose reduction methods for mammography, CT, and positron emission tomography/CT, as well as MR time reduction providing opportunities for faster image acquisition and greater patient throughput [4, 5].
Automated processes to support radiographers in assuring image quality via attenuation correction and technical recall via automated image evaluation are also poised for implementation. Importantly, the opportunities for AI medical imaging augmentation extend to ultrasound where examination quality has for a long time been considered operator, rather than technology, dependent. Sonographers will be able to provide higher-quality ultrasound assessment reports with lower error rates via AI-driven automated fetal measurements and kidney function assessment, as well as image quality assessment [6].
Image processing
The automation of post-processing of CT, MRI, and nuclear medicine studies has been a reality for many years, reducing overall examination time and optimizing patient modality throughput. Newer artificial Intelligence systems in radiology may perform these tasks at even greater speed and scale, potentially allowing for image super-resolution as well as immediate automated segmentation of organs of interest. Early research also promises the possibility of synthetic modality transfer, which is the creation of an MRI image from a CT scan or vice versa, removing the need for a second imaging procedure entirely [7, 8].
Artificial intelligence in radiology has a wide range of applications, from assessment of patients’ reason for the visit to positioning, acquisition, and image processing.
Radiologic Technologists’ Role alongside AI
Without a doubt, the next generation of AI-driven diagnostic imaging tools will have a major influence on radiography practices across modalities as well as on radiographers’ duties and responsibilities. The radiography profession, on the other hand, is accustomed to adapting to new technologies and has always welcomed change, particularly when adequate evidence suggests that such change will ultimately lead to improvement in patient outcomes. As of now, qualitative research outcomes demonstrating clinical impact at scale are limited, but the rate of change and technical advancements in the field of AI suggest that AI-driven solutions will be widely adopted, and radiographers must be prepared for the new opportunities these changes will present while maintaining the core professional value of patient care [9].
The radiography profession must remain patient-facing and patient-focused, and the importance of this aspect of the radiographer role is likely to grow as AI systems are not yet capable of providing human-level conversation and don’t yet possess the level of reassurance and care that patients require from trained health professionals. Radiographers may also have a greater responsibility for advising, defining, and disclosing the radiation risks associated with imaging examinations in accordance with ionizing radiation (medical exposure) regulations and guidance, as well as obtaining patient informed consent, especially if greater automation in referral and vetting processes shortens patient journey times and limits patients’ ability to reflect on the examination referral and discuss any concerns.
Consequently, radiographers now have the opportunity to redefine their roles and develop best practice guidelines for working alongside semi-automated systems, as well as drive forward improvements in patient-facing processes and caregiving time. This may include but is not limited to; developing guides to explain the risks of AI-radiology systems to patients, ascertain the influence of AI processes on human interaction and decision-making, and acquire patient consent for AI research protocols [9].
The radiographers’ role in radiology will be redefined with the advent of artificial intelligence, but remain essential, particularly since AI systems are not yet capable of fully automated human-level conversation and are far from providing the level of reassurance and care that patients require from trained health professionals.
AI-focused Radiology Education and Training
Greater patient throughput, as a result of enhanced automation, will influence personnel requirements. Current work designs that are modality-specific, with minimal cross-modality competence and position flexibility, are unlikely to be sustainable. We should expect all radiographers to develop a range of modality and technology-interfacing abilities as we go towards even bigger patient workloads and continual increases in demand for imaging to enhance disease diagnosis, treatment, and monitoring.
If artificial intelligence (AI) is to become an important component of all imaging approaches in the future, it may be reasonable to expect radiography graduates to have the necessary skills to operate and supervise image acquisition across a wide range of imaging modalities, thereby increasing workforce flexibility. Furthermore, pre-registration radiography programs should guarantee that graduates are familiarized with the principles of AI and its subsets of machine learning and deep learning so that they can confidently engage with them securely and maximize their value. More importantly, these educational changes must be implemented shortly, and healthcare employers must also provide opportunities for existing staff to upgrade their skill sets. Indeed, radiographers must be able to ensure comparative knowledge to support technological adaptation and the necessary changes in work practices and workplace culture [10].
Higher training in the statistical underpinnings of AI systems will also be required to provide radiographers with adequate critical assessment skills for AI outputs in their practice domain. Leadership roles will emerge to change management processes during vendor-specific system deployment and ongoing maintenance. With such a shift in emphasis anticipated in radiology and broader healthcare, education programs must adapt by adding automated technology operation, essential computer science abilities, and technical methods for overseeing and ensuring automated outputs and actions. However, if the radiography profession does not respond to changing technological needs while also looking to the future to redefine clinical roles and responsibilities, there is a real risk of a disconnect between academic departments educating IT competent, multimodality professionals for the future and clinical departments requiring professionals with today’s skills [2].
Radiographers’ training should be updated to include automated technology operation, essential computer science abilities, and technical methods for overseeing and ensuring automated outputs and actions.
AI in Medical Imaging Techniques
AI has demonstrated outstanding accuracy and sensitivity in identifying imaging abnormalities, and it has the potential to improve tissue-based detection and characterization. However, with increased sensitivity comes a significant disadvantage: the identification of tiny changes of uncertain significance.
An analysis of screening mammography, for example, revealed that while artificial neural networks are no more accurate than radiologists in detecting cancer, they consistently have greater sensitivity for pathological findings, particularly subtle lesions. AI may detect image pattern alterations that are difficult for the human eye to recognize. For instance, machine learning can identify tissue alterations indicative of early ischemic stroke within a small time frame from symptom onset with more sensitivity than a human reader [2].
Additionally, AI may provide novel avenues for learning about fine imaging changes that reflect poorly known disease processes. For example, AI could help delineate myocardial tissue changes that reflect inflammation and identify imaging patterns that are highly predictive of treatment response. Using diagnostic AI would help detect MRI patterns related to harsh clinical outcomes, such as severe arrhythmias, hemodynamic instability, and event-specific death, rather than using a non-specific yet broad diagnosis of myocarditis.
High-power quantitative analysis of fine structural image changes might be utilized to predict the likelihood of cancer malignancy and expected tumor dynamics, as well as help modify management approaches. Prostate cancer, for example, although being the most common tumor in males, lacks an efficient screening technique. Multiparametric MRI has been proven to improve the diagnosis of clinically relevant prostate cancer during the last five years, although interobserver variability remains a significant barrier. Numerous deep learning algorithms might improve the evaluation of MRI variables such as texture, volume, and shape, thereby enhancing clinicians’ ability to diagnose advanced prostate cancer while reducing biopsies in low-probability situations [2].
Artificial intelligence diagnostics can improve the image evaluation of texture, volume, and shape, thereby enhancing clinicians’ ability to diagnose pathological findings.
Barriers to AI Implementation in Radiology
To name a few, barriers include the lack of trust and acceptance of the offered systems, the lack of training to enable healthcare practitioners to optimally use the capabilities of AI in radiology, the lack of standardized regulatory frameworks for AI-enabled healthcare products, and the lack of thorough scrutiny of reported studies to ensure a robust knowledge base. It is therefore critical for the design, validation, and adoption of AI that radiographers are kowledgeable, competent, confident, and well-trained to fully materialize the benefits of the new technology while minimizing risks. radiographers also need to be able to explain these benefits and risks to patients; thus, contributing to and sustaining a safe, efficient medical imaging and radiotherapy service, one founded on trust and research evidence [11].
Radiographers should contribute to and sustain a safe, efficient medical imaging and radiotherapy service, one that is based on trust and research evidence on the use of appropriate AI technology.
References
- Hosny, A., et al., Artificial intelligence in radiology. Nat Rev Cancer, 2018. 18(8): p. 500-510
- Rainey, C., et al., Beauty Is in the AI of the Beholder: Are We Ready for the Clinical Integration of Artificial Intelligence in Radiography? An Exploratory Analysis of Perceived AI Knowledge, Skills, Confidence, and Education Perspectives of UK Radiographers. Frontiers in Digital Health, 2021. 3
- Gang, Y., et al., A comparison between manual and artificial intelligence-based automatic positioning in CT imaging for COVID-19 patients. Eur Radiol, 2021. 31(8): p. 6049-6058
- Shi, F., et al., Review of Artificial Intelligence Techniques in Imaging Data Acquisition, Segmentation, and Diagnosis for COVID-19. IEEE Rev Biomed Eng, 2021. 14: p. 4-15
- Clifford, B., et al., An artificial intelligence-accelerated 2-minute multi-shot echo planar imaging protocol for comprehensive high-quality clinical brain imaging. Magn Reson Med, 2022. 87(5): p. 2453-2463
- Ghi, T., et al., Novel artificial intelligence approach for automatic differentiation of fetal occiput anterior and non-occiput anterior positions during labor. Ultrasound Obstet Gynecol, 2022. 59(1): p. 93-99.
- Oren, O., B.J. Gersh, and D.L. Bhatt, Artificial intelligence in medical imaging: switching from radiographic pathological data to clinically meaningful endpoints. The Lancet Digital Health, 2020. 2(9): p. e486-e488
- Nie, D., et al., Estimating CT Image from MRI Data Using 3D Fully Convolutional Networks. Deep Learn Data Label Med Appl (2016), 2016. 2016: p. 170-178
- Oren, O., B.J. Gersh, and D.L. Bhatt, Artificial intelligence in medical imaging: switching from radiographic pathological data to clinically meaningful endpoints. Lancet Digit Health, 2020. 2(9): p. e486-e488
- The Topol Review: Preparing the healthcare workforce to deliver the digital future. 2019; Available from: https://topol.hee.nhs.uk
- Hardy, M. and H. Harvey, Artificial intelligence in diagnostic imaging: impact on the radiography profession. Br J Radiol, 2020. 93(1108): p. 20190840
Disclaimer: The information provided on this website is intended to provide useful information to radiologic technologists. This information should not replace information provided by state, federal, or professional regulatory and authoritative bodies in the radiological technology industry. While Medical Professionals strives to always provide up-to-date and accurate information, laws, regulations, statutes, rules, and requirements may vary from one state to another and may change. Use of this information is entirely voluntary, and users should always refer to official regulatory bodies before acting on information. Users assume the entire risk as to the results of using the information provided, and in no event shall Medical Professionals be held liable for any direct, consequential, incidental or indirect damages suffered in the course of using the information provided. Medical Professionals hereby disclaims any responsibility for the consequences of any action(s) taken by any user as a result of using the information provided. Users hereby agree not to take action against, or seek to hold, or hold liable, Medical Professionals for the user’s use of the information provided.