Helping to Detect Breast Cancer with Artificial Intelligence
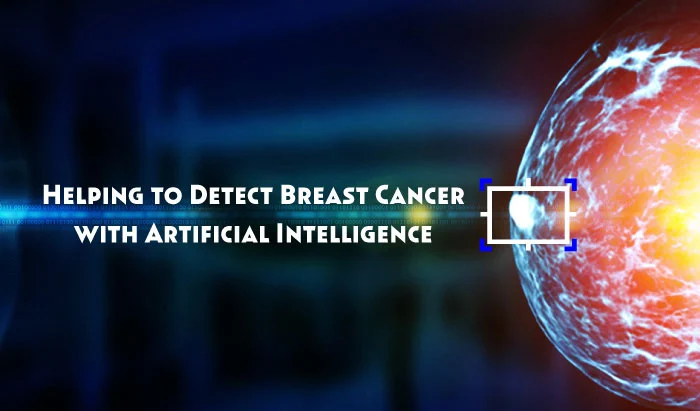
Digital mammography is currently the standard modality for early breast cancer detection, but as with every screening tool, it has its limitations. In an effort to reduce and overcome those limitations, researchers are conducting ongoing studies on the use of artificial intelligence (AI) for the early detection of breast cancer. In a nutshell, the theory of AI can be described as the ability of machines to imitate humans or human brain functions to learn and solve problems. A global effort is being made to develop advanced machine learning algorithms that can accurately interpret screening mammograms. The goal of these algorithms is to potentially improve breast cancer screening by reducing false positives. [1, 2]
So, what does artificial intelligence mean for breast cancer diagnosis? Below, we dig into the current research to answer that question with what researchers in the field know so far.
- A Little Background
- Breast Cancer Prevalence
- Artificial Intelligence: An Overview
- Artificial Intelligence Applications in Mammography
- Applications of AI in Breast MR
- Final Thoughts on Artificial Intelligence and Breast Cancer Diagnosis
- References
A Little Background
Currently in all areas of health care, the primary goal of any clinician in diagnostic imaging is to provide quality patient care while improving workflow and efficiency. This is also true for breast cancer screening and a key driver behind artificial intelligence’s role in the development of breast cancer screening technology.[3] Before the integration of AI, categorization and identification of breast density has always been subjective, which could impact the course of screening and supplemental studies for patients. Clinicians needed to visually assess all images to be able to determine into which of the four categories the breast tissue should be categorized. The Breast Imaging Reporting and Data System (BI-RADS) score was used to assign a score depending on the patient’s breast density—almost entirely fatty, areas of scattered fibroglandular density, heterogeneously dense, and extremely dense. As previously stated, this process is a subjective classification—depending on the judgment of the individual reviewing the images.
In 1956, John McCarthy coined the term “artificial intelligence.” In the more than 60 years since then, AI technology has progressed exponentially, with explosive development over the past 10 years. Today, it continues to grow in its sophistication and applications. In the medical field, AI can be applied to health management, medical imaging, disease screening, clinical decision support, and early disease prediction. It is also used in medical records, literature analysis, and hospital management. AI can be used to analyze medical images and information for disease screening and can assist doctors in making a diagnosis in breast imaging. Al-Antari et al. studied a complete integrated CAD system used for detection, segmentation, and classification of masses in mammography in 2018. This study showed a high accuracy rate of more than 92% in all aspects.[4]
In a study by Rodriguez-Ruiz et al. (2019), 2654 exams were gathered along with readings by 101 radiologists. They used a trained AI system to score the possibility of cancer between 1 and 10. The findings showed that using an AI score of 2 as the threshold could result in a 17% reduction in workload, proving that the AI automatic preselection can significantly reduce the workload of radiologists.[5]
The gist: Artificial intelligence seems to have a high accuracy rate in detecting breast cancer.
Breast Cancer Prevalence
Breast cancer is among the 4 leading cancers in women worldwide (i.e., lung, breast, bowel including the anus, and stomach cancers). It is the leading cause of cancer death among women in developing countries and the second leading cause of cancer death (following lung cancer) among women in developed countries. Developed countries include North America, Europe (including Russia), Australia, New Zealand, and Japan. Those countries in remaining regions are considered developing or emerging nations. These statistics are based on what is known as the Human Development Index (HDI)—a measure of development that considers the standard of living as well as the health and education of the population. In females living in very high and high HDI countries, the two most common cancers will be are breast and colorectal cancers. In medium and low HDI countries, such as India, we find more breast and cervix uteri cancers. While the mortality rates in the US and Canada have declined, mortality rates continue to increase in many countries in Asia, Africa, and Latin America.[6]
International Agency for Cancer Research (IARC) statistics show breast cancer accounts for 25% of all cancer cases diagnosed in women worldwide. Around 53% of these cases come from developing countries, which represent 82% of the world population. The American Cancer Society reported an expected 626,700 breast cancer deaths in 2018 alone.[6] The World Health Organization (WHO) estimates that cancer incidences in general might increase to 27.5 million by 2040, with an estimated 16.3 million deaths expected because of cancer.[6]
The gist: Breast cancer affects women in both developed and developing countries at a disproportional rate and is continuing to increase.
Artificial Intelligence: An Overview
One of the most important aspects to look at in artificial intelligence is called machine learning or ML. Machine learning is a branch of AI and computer science which focuses on the use of data and algorithms to imitate the way that humans learn, gradually improving accuracy. Machine learning algorithms are used to make a prediction or classification.
The field in which large numbers of quantitative features are extracted from medical images and pooled in large-scale analysis to create decision support models is known as radiomics. Radiomics studies follow a methodology of 1) image acquisition, 2) image segmentation, 3) feature extraction, 4) feature selection, and 5) predictive modeling. The methods used at any step of this process may be supervised or unsupervised ML.
ML can be classified in two different categories, unsupervised and supervised. Supervised learning, known as supervised machine learning, is defined by its use of labeled datasets to train algorithms used to classify data or predict outcomes accurately. As input data is fed into the model, it adjusts its weights until the model has been fitted appropriately. This is part of the cross-validation process to ensure that the model avoids overfitting or underfitting.[7]
The terms overfitting and underfitting refer to deficiencies suffered from the model’s performance. Knowing how far off the model’s predictions are can let us know how close it is to overfitting or underfitting. When fitting a model, the goal is to find the “sweet spot” in between underfitting and overfitting. This allows it to establish a dominant trend and apply it broadly to new datasets.
Unsupervised ML classifies the radiomics features without using any information provided or determined by a historical set of imaging data, which is the same kind of data under investigation. Unsupervised learning aims to discover the structure in the data that has no labels or categories assigned to training examples. The most common unsupervised learning task is clustering, which consists of grouping similar examples together according to some predefined similarity metric. The goal is to discover novel patterns in the data, which would otherwise be difficult to notice.
Both radiomics and machine learning tools have been used to predict the likelihood of malignancy and develop imaging biomarkers associated with tumor aggressiveness.
The gist: Machine learning and radiomics play an important part in artificial intelligence and its role in diagnosing breast cancer and other breast pathologies in both MR and mammography.
Artificial Intelligence Applications in Mammography
Currently, breast images are mainly read, analyzed, and diagnosed by radiologists. Due to fatigue caused by a large and long-term workload, images are likely misread causing a misdiagnosis or a completely missed diagnosis. This can be avoided with the use of AI. Computed-aided diagnosis or CAD can be implemented by using a suitable algorithm which can complete the processing and analysis of an image.[8]
AI, either as a detection aid together with a human reader (an advanced form of computer-aided detection) or as an independent second reader, could, in different ways, be used to bypass the limitations of screening mammography. Studies using various algorithms have shown human-like or superhuman performance under conditions that are sometimes fairly realistic.[9]
A team at MIT used a model which was significantly better at predicting risk than existing approaches: It accurately placed 31% of all cancer patients in its highest-risk category, compared to only 18% for traditional models. Rather than manually identifying the patterns in a mammogram, the MIT team trained a deep-learning model to deduce the patterns directly from the data. Using information from more than 90,000 mammograms, the model detected patterns too subtle for the human eye to detect.[10]
We often forget about the time it takes for the mammographer to review the images prior to uploading them to the radiologist. Artificial intelligence is a system efficiency because the AI can take image quality assessment off of mammographers’ plates. So, instead of spending time assessing their own mammograms, mammographers look at the information the AI has provided them, which will tell them their weaknesses and strengths by providing evidence. And if mammographers can spend less time assessing images they can spend more time imaging patients.
Images from mammograms of patients whom the algorithm identified as high risk four years before cancer was diagnosed. (Courtesy of MIT)
Applications of AI in Breast MR
The most sensitive modality for breast cancer detection is magnetic resonance (MR). MR is currently indicated as a supplement to mammography for patients at high risk of developing breast cancer. However, lesion identification can be limited by background enhancement, which may mask or mimic lesions. Additionally, errors in perception, interpretation, and management contribute to false-negative examinations. Like artificial intelligence (AI) tools that increase the diagnostic performance of screening mammography, machine learning tools will likely increase the diagnostic performance of breast MRI by shifting away from subjective and qualitative assessment of images.
According to Dr. Shreyas Vasanawala, PhD, of Stanford University, “when applied during MR image reconstruction, AI can improve image quality or speed up exam times.” What’s more, AI can also detect and correct image artifacts, and perform image processing tasks such as segmentation.
Despite substantial technological development, AI hasn’t been widely adopted by hospitals yet. This delay can be partly attributed to the slow and difficult implementation process with MR. Although the current focus has been on the models themselves, an AI model by itself does not equate to a clinical application at this point.
Screenshot of the QuantX workstation showing a breast MRI for a benign lesion. QuantX combines machine learning and advanced image analysis with a deep database of historical cases to assist radiologists in the assessment and characterization of breast abnormalities on MR images. (Open access)
The gist: In the future, machine learning tools will likely increase the diagnostic performance of breast MRI by shifting away from subjective and qualitative assessment of images.
Final Thoughts on Artificial Intelligence and Breast Cancer Diagnosis
AI, particularly deep learning, is becoming increasingly used in medical imaging and shows excellent performance in medical image analysis tasks. With its advantages of fast computing speed, good repeatability, and no fatigue, AI can provide objective and effective information to doctors and reduce their workload and the rates of missed diagnosis and misdiagnosis. [11] It can allow mammographers additional time during the day to do mammograms and allow them to spend less time assessing their own images. Advances in AI’s use in MR will become apparent in the next few years.
Artificial intelligence in breast imaging is there to assist radiologists and mammography technologists do their job better, not to replace them. The breast imaging team should understand that using this tool will allow them to make their jobs easier and, more importantly, help them to save more lives.
References
- Houssami, N., Lee, C. I., Buist, D., & Tao, D. (2017). Artificial intelligence for breast cancer screening: Opportunity or hype? Breast (Edinburgh, Scotland), 36, 31–33. https://doi.org
- Trister, A. D., Buist, D., & Lee, C. I. (2017). Will Machine Learning Tip the Balance in Breast Cancer Screening?. JAMA Oncology, 3(11), 1463–1464. https://doi.org
- Harvey, S. (2020, May 6). Artificial intelligence & mammography: Where it’s been & where it’s going. Diagnostic Imaging. https://www.diagnosticimaging.com
- Al-Antari, M. A., Al-Masni, M. A., Choi, M. T., Han, S. M., & Kim, T. S. (2018). A fully integrated computer-aided diagnosis system for digital X-ray mammograms via deep learning detection, segmentation, and classification. International Journal of Medical Informatics, 117, 44–54. https://doi.org
- Rodriguez-Ruiz, A., Lång, K., Gubern-Merida, A., Teuwen, J., Broeders, M., Gennaro, G., Clauser, P., Helbich, T. H., Chevalier, M., Mertelmeier, T., Wallis, M. G., Andersson, I., Zackrisson, S., Sechopoulos, I., & Mann, R. M. (2019). Can we reduce the workload of mammographic screening by automatic identification of normal exams with artificial intelligence? A feasibility study. European Radiology, 29(9), 4825–4832. https://doi.org
- American Cancer Society. (2018). Global Cancer: Facts & Figures, 4th edition. http://www.cancer.org
- Cha, K. H., Petrick, N., Pezeshk, A., Graff, C.G., Sharma, D., Badal, A., Badano, A., & Sahiner, B. (2019, March 13). Reducing overfitting of a deep learning breast mass detection algorithm in mammography using synthetic images [Paper presentation]. Proc. SPIE 10950, Medical Imaging 2019: Computer-Aided Diagnosis, 1095004. https://doi.org
- Shenbagavalli, P. & Thangarajan, R. (2018). Aiding the digital mammogram for detecting the breast cancer using shearlet transform and neural network. Asian Pacific Journal of Cancer Prevention, 19(9), 2665–71. doi:10.22034/APJCP.2018.19.9.2665
- Rodriguez-Ruiz, A., Lång, K., Gubern-Merida, A., Broeders, M., Gennaro, G., Clauser, P., Helbich, T. H., Chevalier, M., Tan, T., Mertelmeier, T., Wallis, M. G., Andersson, I., Zackrisson, S., Mann, R. M., & Sechopoulos, I. (2019). Stand-alone artificial intelligence for breast cancer detection in mammography: Comparison with 101 radiologists. Journal of the National Cancer Institute, 111(9), 916–922. https://doi.org
- Yala, A., Lehman, C., Schuster, T., Portnoi, T., & Barzilay, R. (2019). A deep learning mammography-based model for improved breast cancer risk prediction. Radiology, 292(1), 60-66. https://doi.org
- Lei, Y. M., Yin, M., Yu, M. H., Yu, J., Zeng, S. E., Lv, W. Z., Li, J., Ye, H. R., Cui, X. W., & Dietrich, C. F. (2021). Artificial Intelligence in Medical Imaging of the Breast. Frontiers in Oncology, 11, 600557. https://doi.org
- Houssami, N., Kirkpatrick-Jones, G., Noguchi, N., & Lee, C. I. (2019). Artificial Intelligence (AI) for the early detection of breast cancer: a scoping review to assess AI’s potential in breast screening practice. Expert Review of Medical Devices, 16(5), 351–362. https://doi.org
- ICT & Health. (2019, May 13). Using AI to predict breast cancer and personalize care. https://ictandhealth.com
- Reig, B., Heacock, L., Geras, K. J., & Moy, L. (2020). Machine learning in breast MRI. Journal of Magnetic Resonance Imaging, 52(4), 998–1018. https://doi.org
- Sheth, D. & Giger, M.L. (2020). Artificial intelligence in the interpretation of breast cancer on MRI. Journal of Magnetic Resonance Imaging, 51(5), 1310–24. doi:10.1002/jmri.26878
Disclaimer: The information provided on this website is intended to provide useful information to radiologic technologists. This information should not replace information provided by state, federal, or professional regulatory and authoritative bodies in the radiological technology industry. While Medical Professionals strives to always provide up-to-date and accurate information, laws, regulations, statutes, rules, and requirements may vary from one state to another and may change. Use of this information is entirely voluntary, and users should always refer to official regulatory bodies before acting on information. Users assume the entire risk as to the results of using the information provided, and in no event shall Medical Professionals be held liable for any direct, consequential, incidental or indirect damages suffered in the course of using the information provided. Medical Professionals hereby disclaims any responsibility for the consequences of any action(s) taken by any user as a result of using the information provided. Users hereby agree not to take action against, or seek to hold, or hold liable, Medical Professionals for the user’s use of the information provided.
1 Comment